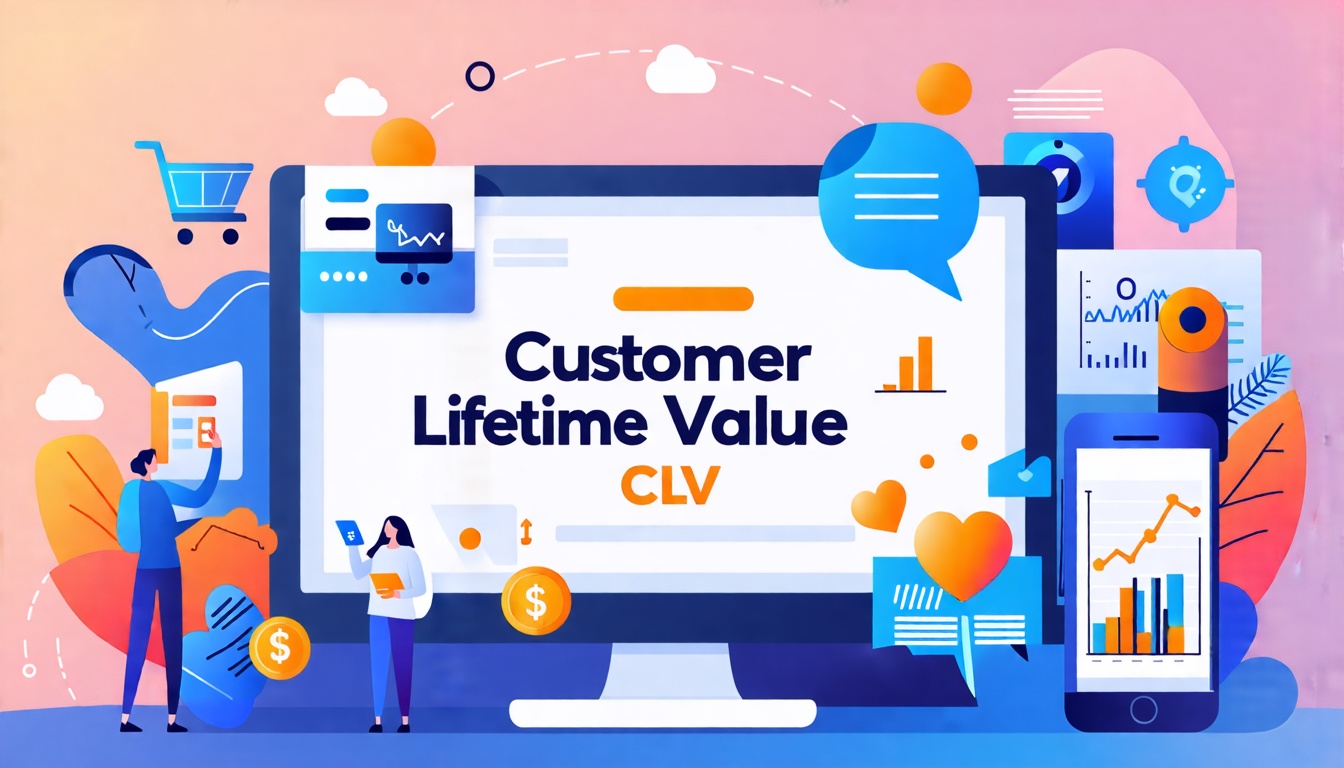
Introduction
Understanding and maximizing Customer Lifetime Value (CLV) has become a cornerstone of success for most B2C businesses today. According to a recent study by Bain & Company, increasing customer retention rates by just 5% can boost profits by 25% to 95%. This staggering statistic underscores the critical importance of CLV in driving long-term profitability and sustainable growth. As financial institutions navigate an increasingly competitive landscape, the ability to accurately measure and leverage CLV has emerged as a key differentiator. By focusing on the right metrics, banks and financial service providers can gain invaluable insights into customer behaviour, preferences, and potential, enabling them to make data-driven decisions that enhance customer relationships and drive business performance. In this comprehensive guide, we will explore six key metrics that are essential for measuring Customer Lifetime Value in the banking and financial services sector. These metrics provide a multifaceted view of customer value, encompassing both financial and behavioural aspects. By mastering these metrics, financial institutions can unlock the full potential of their customer relationships and create strategies that drive long-term success.
Key metrics
Let’s deep dive into 6 crucial metrics relevant for Customer Lifetime Value.
Average Revenue Per User (ARPU)
Definition
Average Revenue Per User (ARPU) is a fundamental metric that measures the average amount of revenue generated by each customer over a specific period, typically annually.
Calculation: To calculate ARPU, divide the total revenue generated in a given period by the number of active customers during that same period.
ARPU = Total Revenue / Number of Active Customers
Relevance
ARPU is a critical metric for financial institutions as it provides a clear picture of the revenue-generating potential of their customer base. It allows banks to segment customers based on their value and tailor strategies accordingly. For example, a retail banking executive might use ARPU to identify high-value customers for premium service offerings or to develop targeted cross-selling campaigns for lower ARPU segments.
Customer Acquisition Cost (CAC)
Definition
Customer Acquisition Cost (CAC) represents the total cost of acquiring a new customer, including marketing expenses, sales efforts, and onboarding processes.
Calculation: CAC is calculated by dividing the total costs associated with acquisition efforts by the number of new customers acquired during a specific period.
CAC = Total Acquisition Costs / Number of New Customers Acquired
Relevance
Understanding CAC is crucial for financial institutions to assess the efficiency of their customer acquisition strategies and ensure that the cost of acquiring customers doesn’t outweigh their potential lifetime value. This metric is particularly important for roles such as Chief Marketing Officers and Customer Experience Directors who are responsible for optimizing marketing spend and improving acquisition processes.
Customer Retention Rate
Definition
Customer Retention Rate measures the percentage of customers who remain with the financial institution over a period, typically annually.
Calculation: To calculate the Customer Retention Rate, use the following formula:
Retention Rate = ((CE – CN) / CS) x 100
Where:
CE = Number of customers at the end of the period
CN = Number of new customers acquired during the period
CS = Number of customers at the start of the period
Relevance
A high retention rate often indicates customer satisfaction and loyalty, which are key drivers of long-term profitability in the financial services sector. This metric is particularly valuable for Customer Success Managers and Relationship Bankers who are focused on building and maintaining strong customer relationships.
Net Promoter Score (NPS)
Definition
Net Promoter Score (NPS) is a widely used metric that measures customer loyalty and satisfaction by asking customers how likely they are to recommend the financial institution to others.
Calculation: NPS is calculated by subtracting the percentage of detractors (those who give a score of 0-6) from the percentage of promoters (those who give a score of 9-10) on a scale of 0-10.
NPS = % Promoters – % Detractors
Relevance
While not a direct financial metric, NPS is a powerful indicator of customer satisfaction and loyalty, which are closely linked to CLV. It provides valuable insights for executives across the organization, from Product Managers looking to improve offerings to Customer Experience Officers aiming to enhance overall service quality.
Cross-Sell Ratio
Definition
The Cross-Sell Ratio measures the average number of products or services a customer uses within the financial institution.
Calculation: To calculate the Cross-Sell Ratio, divide the total number of products or services sold by the total number of customers.
Cross-Sell Ratio = Total Products/Services Sold / Total Number of Customers
Relevance
A higher cross-sell ratio typically indicates deeper customer relationships and higher potential CLV. This metric is particularly important for Product Managers and Sales Directors in developing strategies to increase product adoption and maximize customer value.
Customer Profitability Score
Definition
The Customer Profitability Score is a comprehensive metric that takes into account all revenues generated by a customer minus the total costs associated with maintaining that customer relationship. While the specific calculation can vary based on the institution’s cost allocation methods, a basic formula is:
Customer Profitability = Total Customer Revenue – (Direct Costs + Allocated Indirect Costs)
Relevance
This metric provides the most holistic view of a customer’s value to the institution, incorporating both revenue generation and cost considerations. It’s particularly valuable for C-suite executives and Financial Analysts in making strategic decisions about resource allocation and customer management strategies.
Evolving trends in banking CLV measurement
The landscape of banking and financial services is undergoing a seismic shift, and with it, the process of measuring Customer Lifetime Value (CLV) is evolving at breakneck speed. As we peer into the crystal ball of finance, it’s clear that the future of CLV metrics is inextricably linked with technological advancements and changing consumer behaviors.
First and foremost, the rise of big data and advanced analytics is revolutionizing how banks calculate and interpret CLV. Gone are the days of simple transactional analysis; today’s financial institutions are diving deep into a sea of data points, from social media interactions to geolocation data, to paint a more holistic picture of customer value. This trend is set to intensify, with AI and machine learning algorithms becoming increasingly sophisticated in predicting future customer behaviors and potential lifetime value. Moreover, the proliferation of digital banking channels dramatically alters the customer journey. Banks gain unprecedented visibility into customer preferences and habits as more transactions and interactions move online. This digital footprint allows for real-time CLV calculations, enabling banks to make on-the-fly decisions about customer engagement and personalized offerings.
Another significant trend is the integration of non-financial data into CLV models. Banks are beginning to recognize that a customer’s value extends beyond their account balance. Factors such as social influence, brand advocacy, and even environmental consciousness are woven into CLV calculations, providing a more nuanced understanding of a customer’s true worth to the institution. CLV models of the future will need to account for a customer’s value across a wide range of interconnected services, from mortgages to investment portfolios to insurance products. Lastly, regulatory changes and increased focus on customer privacy are shaping how banks collect and utilize data for CLV calculations. The future will likely see a delicate balance between leveraging comprehensive customer data and respecting individual privacy rights, potentially leading to more transparent and customer-centric CLV models. As these trends converge, they’re not just changing how CLV is measured but fundamentally altering what it means to be a valuable customer in the banking sector. Financial institutions that can adapt to these changes and harness the power of advanced CLV metrics will be well-positioned to thrive in the increasingly competitive and complex world of modern banking.
The Fosfor Decision Cloud: Shaping banking’s future
The banking and financial services landscape is evolving daily, and the Fosfor Decision Cloud emerges as a game-changing solution poised to reshape the industry’s future in such a turbulent environment. It offers a robust suite of tools to revolutionize how banks approach Customer Lifetime Value (CLV) and the decision-making processes.
At its core, the Fosfor Decision Cloud harnesses the power of advanced analytics, machine learning, and artificial intelligence to provide financial institutions with unprecedented insights into their customer base. By aggregating and analyzing vast amounts of data from diverse sources, the platform offers a 360-degree view of each customer, far surpassing traditional CLV models in depth and accuracy.
The platform’s predictive analytics capabilities are another game-changer for the banking sector. By leveraging sophisticated algorithms, the Fosfor Decision Cloud can forecast future customer behaviors, identify potential churn risks, and uncover hidden opportunities for cross-selling and upselling. This forward-looking approach allows banks to proactively engage with customers, enhancing satisfaction and loyalty while maximizing lifetime value.
The platform’s emphasis on explainable AI is particularly relevant in the highly regulated banking environment. As CLV models become more complex, the ability to understand and justify decisions based on them becomes crucial. The Fosfor Decision Cloud provides transparent, interpretable insights, helping banks maintain regulatory compliance while leveraging the power of advanced analytics.
Furthermore, the scalability and flexibility of the Fosfor Decision Cloud make it an ideal solution for banks of all sizes. Whether a local credit union or a multinational banking corporation, the platform can be tailored to meet specific needs and grow alongside the institution. As we look to the future, the Fosfor Decision Cloud is set to play a pivotal role in shaping how banks approach customer relationships, risk management, and strategic decision-making. Providing a comprehensive, data-driven foundation for CLV calculations and beyond empowers financial institutions to navigate the complexities of modern banking with confidence and agility.
The Fosfor Decision Cloud represents more than a technological upgrade – it’s a paradigm shift in how banks understand and interact with their customers. As the financial services landscape evolves, this innovative platform stands ready to guide banks into a future where data-driven insights, personalized customer experiences, and agile decision-making are the keys to success. For any financial institution looking to stay ahead of the curve and maximize the lifetime value of their customer relationships, the Fosfor Decision Cloud is not just a product, but a strategic imperative.
Conclusion: Harnessing the power of CLV metrics
As we’ve explored, these six key metrics—Average Revenue Per User, Customer Acquisition Cost, Customer Retention Rate, Net Promoter Score, Cross-Sell Ratio, and Customer Profitability Score—form a powerful framework for measuring and maximizing Customer Lifetime Value in the banking and financial services sector. By integrating these metrics into their strategic decision-making processes, financial institutions can comprehensively understand their customer relationships, identify growth opportunities, and optimize their operations for long-term success.
As we look to the future, the importance of CLV measurement in financial services is only set to increase. Emerging technologies such as artificial intelligence and machine learning enable even more sophisticated approaches to CLV analysis, allowing for real-time insights and predictive modeling.
To stay competitive in this rapidly evolving landscape, C-suite executives must prioritize implementing these CLV metrics in their organizational strategies. By doing so, they can ensure their institutions are well-positioned to build stronger customer relationships, drive sustainable growth, and navigate the challenges of an increasingly digital and customer-centric financial world.
Remember, the journey to maximizing Customer Lifetime Value is ongoing. It requires a commitment to data integration, leveraging the right technologies, and fostering a customer-centric culture throughout the organization. The Fosfor Decision Cloud can be crucial in this journey, helping financial institutions transform raw data into actionable insights and democratize access to these critical CLV metrics across decision-makers. By embracing these metrics and the technologies that support them, financial institutions can unlock the full potential of their customer relationships, driving growth, profitability, and long-term success in an increasingly competitive market.