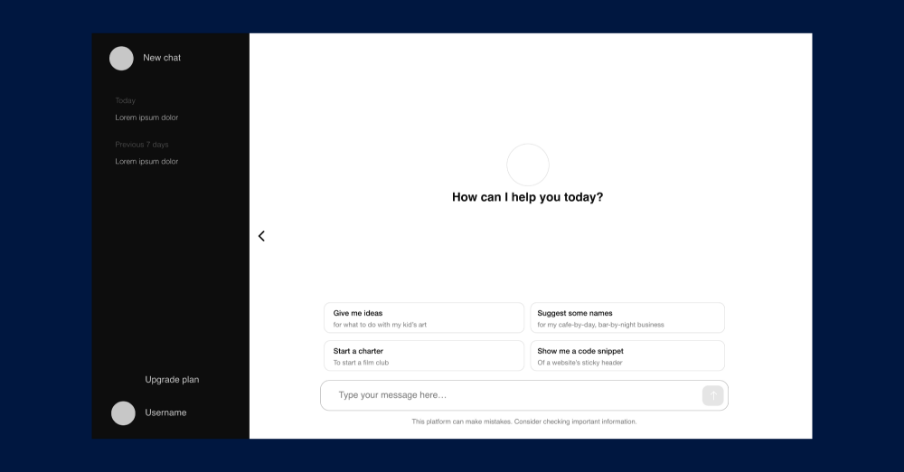
Prompt Engineering: The New Era of AI
Trends are changing in the data science domain every day. Many tools, techniques, libraries, and algorithms are developing daily. This constantly changing landscape keeps the data science domain at the bleeding edge. The techniques and methods used to solve different tasks in Machine Learning (ML)/ Deep Learning (DL) and Natural Language Processing (NLP) are also changing.
What is next in Artificial Intelligence (AI)? This is one of the questions every data science aspirant should ask themself. In the initial years of the AI revolution, symbolic AI-related rule-based systems solved the different ML/DL or NLP problems such as text classification, natural language text extraction, passage summarization, data mining, etc. As time passed, statistical models replaced symbolic AI-based ML solutions. In statistical models, we use machine learning or deep learning systems with lots of training data and use model prediction. Statistical models need lots of training data for training. Realistically, obtaining that much labeled, cleaned data to train isn’t easy. This significant limitation to statistical modeling was overcome by a new learning method called prompt engineering.
Prompt-based machine learning, aka prompt engineering, has already opened many possibilities in ML. Lots of research is taking place in this area globally. Let’s dive into prompt engineering and why it is becoming more popular in data science.
What is Prompt Engineering?
Prompt engineering is the process of designing and creating prompts, or input data for AI models to train on as they learn specific tasks.
Prompt engineering is a new concept in artificial intelligence, particularly, in NLP. In prompt engineering, the task description is embedded in the input.. Prompt engineering typically works by converting one or more tasks into a prompt-based dataset and training a language model with what has been called “prompt-based learning” or just “prompt learning.”
This technique became popular in 2020 with GPT- 3, the third generation of Generative Pretrained Transformer. AI solution development has never been simple, but with GPT-3, you only need a meaningful training prompt written in simple English.
The first thing we must realize while working with GPT-3 is how to design required prompts according to our use case. Like In statistical models, the quality of training data boosts prediction quality. Here, the quality of input prompts increases the quality of the task it performs. So, a good prompt can deliver a good task performance in the desired favorable context. Writing the best prompt is often a matter of trial and error.
Prompt-based learning is based on Large Language Models (LLM) that directly model text probability. In contrast, traditional supervised learning trains a model to take an input x and predict an output y as P(y|x). In order to use these models for prediction tasks, the initial input (x) is changed using a template into a textual string prompt with some empty slots (x’). The language model is then used to probabilistically fill the empty information to obtain a final string (x^), from which the final output (y) can be deduced. This architecture is effective and appealing for various reasons. For example, this architecture allows the user to pre-train the language model on enormous volumes of unlabeled text and conduct few-shot, one-shot, or even zero-shot learning by specifying a new prompting function.
Pretrain – Prompt – Predict Paradigm
As you now know, prompt engineering has evolved with the pretrained LLMs. As mentioned above, prompt engineering is based on the LLM. So all the prompts we trigger are hit on a Language Model behind the scene. A powerful, well-trained language model should be selected/Identified before we start the prompt engineering. The first step is selecting the pre-trained model.
Pretrain
While selecting a pre-trained model, considering the pretraining objective is significant. One can pretrain a language model in multiple ways depending on the context.
Pretraining via next token prediction
Next-token prediction is when we simply want to predict the next word after giving all the previous words in context. This is one of the most straightforward and easy-to-understand pretraining strategies. These pretraining objectives are so important for prompting considerations because they will affect the types of prompts we can give the model and how we can incorporate answers into those prompts.
Pretraining via masked token prediction
Instead of predicting the next token by taking all other previous tokens, the masked token prediction method predicts any masked tokens within the input, given all the surrounding contexts. The classic BERT language model has been trained via this strategy.
Training an entailment model
More than a pretraining strategy, the entailment helps in the prompted classification tasks. Entailment means, given two statements, we want to determine if they imply one another, conflict with one another, or are neutral, meaning they have no bearing on one another.
Prompt and Prediction
Once we select the pretrained language model, the next step is designing the appropriate prompt for our desired task. Understanding what the pretrained language model knows about the world and how to get the model to use that knowledge to produce beneficial and contextual results is the key to generating successful prompts.
Here, in the form of a training prompt, we provide the model with just enough information to enable it to recognize the patterns and complete the task at hand. We don’t want to overwhelm the model’s natural intelligence by providing all the information simultaneously.
Given that the prompt describes the task, picking the right prompt significantly impacts both the accuracy and the first task that the model does.
As a general guideline, we should strive to elicit the required response from the model in a zero-shot learning paradigm when developing a helpful prompt. This indicates that the task should be finished without undergoing any sort of fine-tuning. If, after receiving the model’s response, you believe it falls short of your expectations, give the model one specific example along with the suggestion. The One-Shot Learning paradigm is used to describe this. If you still believe that the responses do not satisfy your needs, give the model a couple of additional instances along with the request. The Few-Shot Learning paradigm is what is used in this.
For simplicity, the standard flow for training prompt design should look like this: Zero-Shot → One Shot → Few shot
Let us check how we can generate prompts on the GPT-3 language model to perform our custom tasks.
Prompt with Zero-Shot
Consider the domain identification problem in NLP. In statistical modeling, we are used to solving it by training a machine learning or deep learning model using extensive training data. Modeling and training are not feasible in statistical machine learning without significant time and proper technical knowledge.
We can identify domains of some famous personalities using prompts in GPT-3 with zero-shot learning. Since the setup and background of GPT-3 are beyond this topic, we are directly moving to generally understanding prompts.
Prompt:
The following is a list of celebrities and the domains they fall into:
Leonardo DiCaprio, Elon musk, Ishan Kishan, Kamala Harris, Andrey Kurkov
Response:
Looks amazing right? Without providing prior knowledge, the pretrained model detects each domain based on the prompt.
Prompt with One-Shot
Consider the question-answering problem. Question-answering is one of the fundamental problems in NLP. Others include word sense, disambiguation, named entity identification, anaphora and cataphora resolution. Training a question-answering model from scratch and ensuring its performance is very complex in traditional machine learning.
Question-answering in machine learning is complex because it requires the model to understand natural language and context, reason, and retrieve relevant information from a large amount of data. Furthermore, natural language is complex and ambiguous, with multiple meanings and interpretations for the same words or phrases. This makes it challenging to accurately understand the question’s intent and provide an appropriate answer. The model must handle various forms of language, such as idioms, colloquialisms, and slang.
Context is another important factor in question-answering. The meaning of a word or phrase can vary depending on the context in which it is used. For example, the word “bat” can refer to a flying mammal or a piece of sports equipment, depending on the context. Thus, the model needs to be able to understand the broader context of a question to provide a relevant answer
Let us see how we can resolve this problem with prompt engineering.
An example and input are distinguished with the token
Prompt:
Context: The World Health Organization is a specialized agency of the United Nations responsible for international public health. Headquartered in Geneva, Switzerland, it has six regional offices and 150 field offices worldwide. The WHO was established on 7 April 1948.