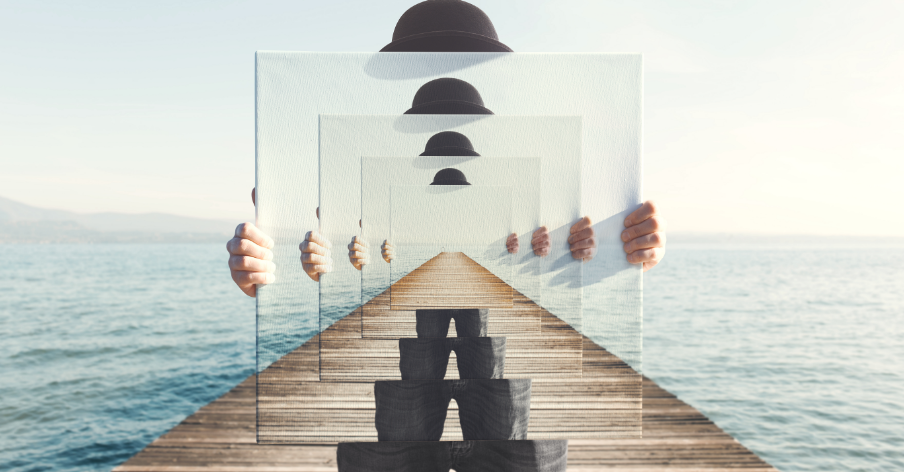
Usually, Machine Learning (ML) based experimentation follows the 80/20 rule. 80% of the time spent by Data scientists is relegated to finding, cleaning, and organizing data, while the rest, only 20%, goes into analyzing or experimenting. For this reason, if one plans to publish an experiment using research papers as a baseline, obtaining similar results for learning operations can be challenging when figuring out scoring or performance.
This raises the question: “Is the given ML use case reproducible or not?” Let’s understand how it can be. The boom in ML adoption has brought with it a rise in skepticism surrounding AI use cases. Researchers are trying to make ML more transparent through Explainable AI (XAI). However, without reproducing ML experiments, one cannot assume the results to be credible.
Importance of Reproducibility
Reproducibility in machine learning can be generally defined as an internal process in which experimental data testing moves from exploratory to larger, more controlled experimental trials that will run multiple times. The goal is to have the results:
- Merge into a larger data set
- Have a larger sample size
- Have high statistical power
These factors make machine learning operations and experiments worthy of published research reports so other data scientists may attempt to replicate findings. However, unfortunately, this is rarely happening for several reasons.
Indefinite and uncontrollable study variations can deviate the model output and result in reproducibility failure. Some of these variations include but are not limited to basic changes in data or different package versions and environment setup (dev, test, prod, etc.).
With better Reproducibility, we address the problem of experimentation variations. In general, it reduces the probability of experimentation error and makes it more reliable and scalable. It helps make the model yield similar results irrespective of the number of times we run the same experiment. This gives the reproducible ML experiments stability and makes them more robust towards their environment, software, and data sensitivity. Furthermore, it helps make the experimentation more seamless across different model development and deployment stages.
Lack of trustworthiness in use cases leads to holes in a few crucial aspects of ML experimentation. These include the following four pillars.
- 1. Authenticity – If we get different results employing the same experimentation, this puts a big question mark on the authenticity of the complete process.
- 2. Dependability – With the adoption of ML operations in our day-to-day lives, the dependency on ML is enormous. Hence if we cannot rebuild or explain the use case, we cannot be dependent on it. The baseline for end users is to have more transparent and reliable ML systems. As discussed in Section’s article about the significance and challenges of reproducibility in machine learning, we cannot trust the results if we cannot reproduce a model, thus reducing the model’s dependability.
- 3. Benchmark – This means a standard guideline that can be used for comparisons. If we want to improve our reproducible ML model, we need some benchmarking of the earlier model to compare with. But even before starting the new experiment, if we cannot reproduce the earlier model with similar results, it will be unethical to vouch for an improved model version.
- 4. Accelerated experimentation – Once we have a standard approach designed for the investigation that works, it makes it more secure to perform similar experiments and helps to accelerate the model experimentation time. This also forms a base for a Continuous Improvement and Continuous Delivery (CI/CD) pipeline. CI/CD is an agile DevOps workflow focused on a frequent and reliable software delivery process.
Fig 1 – Benefits of Reproducible ML
Challenges with Reproducible ML
As stated, data scientists often try to replicate ML papers, but most of us end up with a different result set. The solution is reliable reproducibility in machine learning. Still, a few challenges highlighted below hamper ML experiments, including issues within ML operations and processes.
No trace of historical experiments
The biggest challenge in reproducing ML experiments is the lack of records for past investigations. If one doesn’t log or store the input data, it becomes cumbersome to achieve similar results. This is the case, especially if no logging happened with:
- Model training
- Test data used for validation
- Package versions used in the code
- Code copy
- R/Python language version
- Env variables, etc.
- Hyperparameter values used
- Initialization or randomization values used during experimenting
Understanding what and when to log
When dealing with ML use cases, capturing data, code, model, output, environment, and other external variables is mandatory to support a reproducible ML use case. Some team members are often not equipped to handle the logging scenario and miss recording all the necessary artifacts. Decisive Edge emphasizes that one should proceed with ML use case experimentation while keeping reproducibility in mind across each development and deployment life cycle lifecycle stage.
How to achieve Reproducibility
The primary purpose of reproducibility is to reduce the variations in the model behavior when the model moves through different stages from dev to production. To help this, capturing and maintaining the changes in data, code, model, and environment across locations is very crucial.
There are multiple artificial intelligence tools in the market to help track and maintain these components individually. Here are some examples:
Data
Data can be stored using data version tools like DVC, Delta Lake, Git LFS, etc. DVC is a data and ML experiment management tool that takes advantage of existing engineering toolsets like Git and CI/CD.
Code
Code can be version-controlled using Source Code Management tools like Git, SVN, TFS, etc. Version-control helps to keep track of the changes in the code so that if something goes wrong, we can make comparisons in different code versions and revert to any previous version that we want.
Environment
It’s almost impossible to reproduce models without being able to match the same environment and software libraries/frameworks the model was built on. ML frameworks are constantly being upgraded, so storing and keeping the information on the software version and the environment used in building the model is a must. Tools such as Conda, Docker, Kubeflow, Pipenv, etc., can help us achieve this.
Model Artifacts
Model artifacts consist of:
- All the model metadata
- Datasets used for training
- Model outputs
- Model logs
- Metrics used to evaluate
- Model monitoring logs and more
The artifacts help to compare the model with a baseline to check the Model metrics and performance. Neptune and ML Flow can help us store these artifacts in the centralized registry.
Fig 2 – ML Reproducibility in Refract