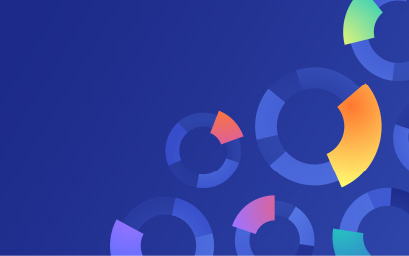
The story of risk assessment so far: A recap
The evolution of underwriting and risk assessment in insurance has been fascinating. In the early 1900s, underwriting relied heavily on intuition and manual records. By the mid-20th century, the incorporation of actuarial science introduced statistical models, enhancing accuracy. The 1980s marked the advent of computer technology, revolutionizing data collection in the field and laying the groundwork for future data analytics. With the rise of big data and machine learning for insurance(ML) in the 2000s, risk assessment is gradually becoming more sophisticated. Today, AI and advanced analytics are heralding real-time risk evaluation and personalized policies proposing an industry shift towards data-driven decision-making. (Haueter)
The imminent need for a comprehensive data-to-decisions capability
Today in the insurance space, consistent underwriting profitability in both hard and soft markets is becoming a necessity while weathering increasing natural catastrophe (NATCAT) events(Swiss Re Group, 2024). In such times, the adoption of data and analytics practices are increasingly becoming imperative for differentiation in pricing and risk selection. Data science enhances underwriting by integrating historical and real-time data, leveraging machine learning algorithms to classify risks and map exposures more accurately, and using predictive analytics to identify potential losses(Institute and Faculty of Actuaries, 2017). This enables insurers to refine pricing models and optimize risk selection, thus ensuring a competitive advantage(Javanmardian, Srivastava, Talischi, & Cameron, 2021).
When the machines start to learn: Machine Learning for Insurance
Machine learning for insurance(ML) models are revolutionizing the underwriting and risk assessment processes. For instance, insurers use ML to analyse vast amounts of data from various sources- internal sources such as historical claims, customer data, or external sources such as weather patterns, and social media data, to predict potential risks (whether physical or moral) more accurately. Predictive analytics models help underwriters identify risk profiles so that they may be underwritten at the right price, terms and conditions.
ML Models can simulate claims based on risk improvement and mitigation factors, capacity availability and other evolving trends. In addition, real-time data from IoT devices, such as telematics in vehicles, allows insurers to adjust premium offerings based on actual driving behaviour enhancing risk pricing, or provide usage-based premiums enhancing customer satisfaction. Combining the pattern detection of ML models along with simulation would help in identifying exposures to different grades of natural catastrophe (NATCAT) events in terms of both frequency and severity, which would help in estimating the extent of CAT reinsurance needed. These applications of ML enable underwriters to make more informed, data-driven decisions, ultimately improving profitability and risk management. (Van Dalen, Cusick, & Ferris, 2021)
The artificial intelligence outlook: Future capabilities
Adding to this, rule-based AI models can also generate or draft risk-specific terms and conditions for an underwriter’s review. In theory, Generative AI in insurance (GenAI)could use be used to guide product or rider development as per changes in risk or underwriting cycle (Figure 1: The Underwriting cycle) conditions. It could also be used in automating parts of health underwriting by interpreting imaging and patient health records. Generative AI in Insurance will one day be used to automate risk assessments by generating risk scores base on external data sources. For example, cargo insurance risk scores based on blockchain technology, tracking possible privacy activities on shipping routes.
In short, the breadth and depth of experience required by an underwriter can be knowledge transferred through ML and GenAI models. Moreover, these techniques can also disseminate acceptance strategies and monitor underwriting guideline compliance on various granular levels.
Insights to decisions: Getting to production
When scaling AI/ML models into production, several things can go wrong, highlighting the need for MLOps (Machine Learning Operations). For example, a model trained on historical data might fail to adapt to recent, unseen data, leading to inaccurate predictions and financial losses. Imagine an insurer deploying a model to assess auto insurance claims. If the model isn’t updated with recent driving data, it might misclassify safe drivers as high-risk, upsetting customers and increasing churn.
Operational issues like model latency can also arise. A delay in processing real-time data from IoT devices could hinder timely risk assessment, affecting customer service. Without MLOps, managing these issues can be challenging, as it ensures continuous integration and delivery, automated monitoring, and seamless updates, maintaining model accuracy and reliability in production.
Another scenario involves data drift, where the statistical properties of the input data change over time leading to degradation in model performance. For instance, in property insurance, if the model isn’t retrained to consider the latest climate data, it might underestimate the risk of natural catastrophes, resulting in inadequate premiums and increased claims costs. This would be a concept drift. Another type of drift is population drift where the change occurs in the composition of the population or the data sample. For example, with bettering risk selection the population will become class imbalanced towards good risks. Then there is seasonal drift that occurs with predictable and periodic changes in the data. These changes are often cyclical like the hardening and softening of capacity as per Figure 1.Other factors that can cause data drifts of various kinds can be regulatory changes, reinsurance treaty changes, risk mitigation features becoming the norm or statutory requirements, evolving trends.(Gomede, 2024)
With any kind of data drift there is a requirement of reevaluating the models as they may require to be retrained on new unseen data. Alternatively, adaptive learning techniques may be used to automatically adjust to changes in data over time. This requires continuous or periodical monitoring of the reliability and accuracy of predictive models.
The integration of MLOps (Machine Learning Operations) is essential for every insurance and risk management data strategy. MLOps ensures the seamless deployment, monitoring, and maintenance of ML models, addressing critical challenges such as data drift, model degradation, and operational inefficiencies. Without MLOps, insurers risk deploying outdated or inaccurate models, leading to poor risk assessments and financial losses. Moreover, MLOps facilitates continuous model improvement by automating retraining processes and incorporating new data streams, thus maintaining model accuracy and relevance. This is crucial in a dynamic environment where factors like climate change and evolving consumer behaviors continually alter risk landscapes. By adopting MLOps, insurers can enhance predictive accuracy, optimize pricing models, and deliver superior customer service, ultimately driving profitability and competitiveness in the market.
Enabling the average Underwriter powered by The Fosfor Decision Cloud
The Fosfor Decision Cloud empowers insurers to scale their underwriting and risk assessment processes by seamlessly integrating advanced analytics into the data-to-decisions journey. By leveraging this platform, insurers can automate data ingestion, apply sophisticated machine learning models, and derive actionable insights in real time. This capability is crucial for adapting to rapidly changing risk environments, such as evolving climate patterns and shifting market conditions. Fosfor’s robust MLOps framework ensures continuous model monitoring and retraining, maintaining accuracy and reliability. This enables insurers to make precise, data-driven decisions, optimize pricing strategies, and enhance customer satisfaction, ultimately driving profitability and competitive advantage in the insurance sector.
References
Gartner Press Release. (2024, April 25). Gartner Identifies the Top Trends in Data and Analytics for 2024. Mumbai, India: Gartner.
Gomede, E. (2024, January 11). Understanding Types of Data Drift in Machine Learning. 𝐀𝐈𝐦𝐨𝐧𝐤𝐬.𝐢𝐨. Retrieved from www.medium.com
Haueter, N. V. (n.d.). A History of Insurance. Retrieved from www.swissre.com
Institute and Faculty of Actuaries. (2017, September). Data Science in Insurance: Opportunities and Risks for Consumers . Retrieved from www.actuaries.org.uk
Javanmardian, K., Srivastava, A., Talischi, C., & Cameron, S. (2021, September). How data and analytics are redefining excellence in P&C underwriting. p. 8. Retrieved from www.mckinsey.com
Swiss Re Group. (2024, March 26). New record of 142 natural catastrophes accumulates to USD 108 billion insured losses in 2023, finds Swiss Re Institute. 5. Zurich: Swiss Re Institute. Retrieved from www.swissre.com
Van Dalen, B., Cusick, K., & Ferris, A. (2021, February 24). The rise of the exponential underwriter. Deloitte Insights, p. 24. Retrieved from www2.deloitte.com